The role of machine learning in credit scoring systems
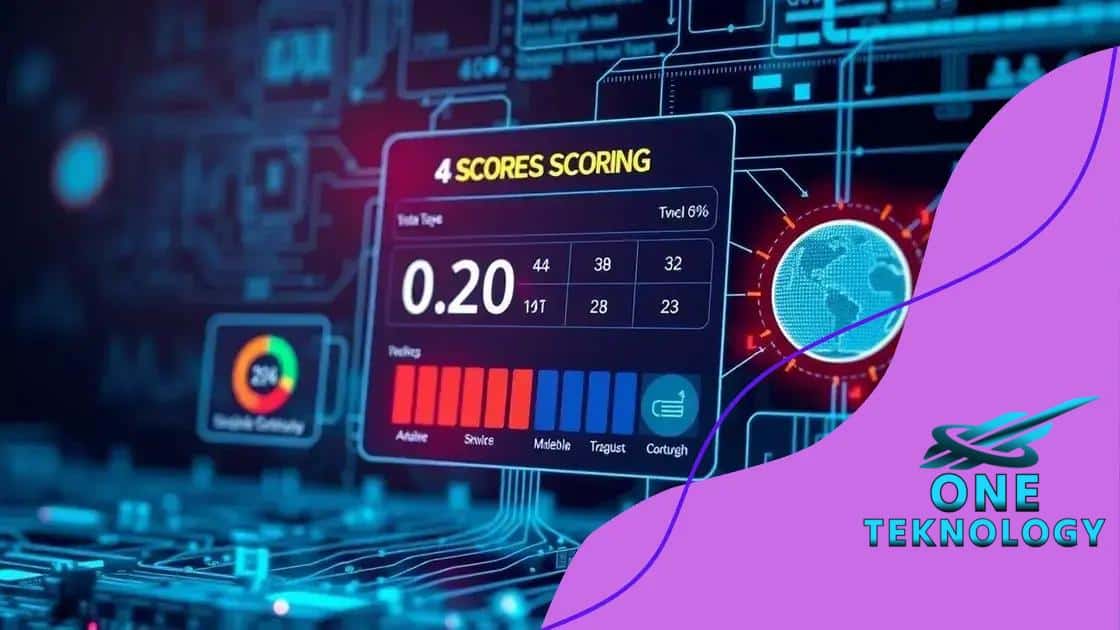
The role of machine learning in credit scoring systems enhances accuracy and fairness by analyzing vast data to better assess borrower risk and predict behavior.
The role of machine learning in credit scoring systems is changing the way lenders assess risk and make decisions. Curious about how this technology reshapes finance?
Understanding credit scoring systems
Understanding credit scoring systems is essential in today’s financial landscape. These systems play a significant role in determining how lenders assess potential borrowers. A credit score can impact interest rates and loan approval, making it crucial for consumers to comprehend how these scores are calculated.
What is a credit scoring system?
A credit scoring system is a mathematical model that evaluates a borrower’s creditworthiness. It analyzes various factors, including past credit history, outstanding debts, and payment behavior. Each element contributes to a numerical score, often ranging from 300 to 850.
Key factors affecting credit scores
Several factors significantly influence credit scores:
- Payment history: Timely payments boost scores, while missed payments can harm them.
- Credit utilization ratio: Keeping balances low compared to credit limits is beneficial.
- Length of credit history: Longer credit histories tend to be favorably viewed.
- Types of credit: A mix of credit types, such as revolving and installment accounts, can improve scores.
These components are weighted differently. For instance, payment history usually has the most significant impact, while the type of credit may hold less weight. Understanding these factors empowers consumers to manage their credit effectively.
Moreover, credit scoring systems can differ among various lenders. Some might use proprietary algorithms, while others rely on widely accepted models like FICO or VantageScore. This variation underscores the importance of being knowledgeable about one’s credit standing and monitoring it regularly.
By regularly checking your credit reports, you can identify areas for improvement. This proactive approach can lead to better credit scores, which opens doors to favorable lending opportunities.
The impact of machine learning on accuracy
The impact of machine learning on accuracy in credit scoring systems is revolutionary. By utilizing advanced algorithms, machine learning increases precision in assessing borrowers’ profiles. This enhancement leads to better decision-making for lenders, minimizing risks associated with lending.
How machine learning improves accuracy
Machine learning analyzes vast amounts of data more efficiently than traditional methods. It identifies patterns and trends that are often overlooked by human analysts. This capability allows lenders to create more tailored credit assessments.
Key advantages of machine learning
Some key benefits include:
- Enhanced predictive power: Algorithms can predict borrower behavior based on historical data.
- Real-time analysis: Machine learning enables continuous monitoring of credit data.
- Customized scoring models: Lenders can tailor models to fit specific demographics or risk profiles.
- Reduction of human bias: By relying on data, machine learning minimizes subjective judgments.
As machine learning continuously learns from newly available data, it becomes increasingly effective in identifying creditworthy individuals. This adaptability is crucial in today’s fast-changing financial landscape, where borrower characteristics can quickly evolve.
Moreover, the integration of machine learning allows for the identification of fraud and anomalies, which traditional scoring methods might miss. Transparency in scoring processes can lead to increased trust from consumers as well.
How machine learning improves risk assessment
Machine learning significantly enhances risk assessment in credit scoring systems. By analyzing vast amounts of data, it identifies patterns and correlations that traditional methods might overlook. This ability leads to more accurate evaluations of a borrower’s likelihood to default.
Data analysis and pattern recognition
Machine learning algorithms excel in processing large datasets. They can identify trends or anomalies in credit histories that contribute to a more comprehensive understanding of a borrower’s risk profile. This depth of analysis helps lenders make better-informed decisions.
Types of machine learning techniques
Several machine learning techniques are particularly effective in risk assessment:
- Supervised learning: This technique uses labeled data to train models that predict future outcomes based on historical patterns.
- Unsupervised learning: This approach identifies hidden patterns in data without predefined labels, uncovering insights about different borrower segments.
- Neural networks: These systems mimic the human brain’s neural connections and are excellent at recognizing complex patterns in data.
- Decision trees: These tools help model decisions and associated risks in a visually intuitive format.
As these algorithms learn from new data, their predictive abilities improve over time. This learning process ensures that risk assessments are always updated and reflective of current trends in borrower behavior.
Additionally, machine learning can help uncover subtle signs of potential risk that may not be evident to human analysts. Factors such as spending habits, online behavior, and even social media data can be included in risk evaluations, leading to a more rounded perspective of a borrower’s financial reliability.
Ethical considerations in credit scoring
Ethical considerations in credit scoring are essential to ensure fairness and transparency in lending practices. As these systems increasingly rely on data, it’s crucial to recognize how biases can affect outcomes. The integration of machine learning brings significant benefits, but it also presents ethical challenges.
Bias in data
One major concern is bias in the training data used for credit scoring models. If historical data reflects discrimination or unfair practices, machine learning algorithms may perpetuate these biases. For instance, certain demographics may appear less creditworthy due to past systemic inequalities.
Transparency and accountability
Another ethical consideration is the need for transparency in credit scoring processes. Consumers deserve to understand how their scores are determined. Key factors include:
- Access to information: Borrowers should have the right to access their credit scores and know how they were calculated.
- Clear explanations: Lenders must provide clear explanations about why a certain score was given.
- Recourse for disputes: There should be a process for consumers to dispute errors in their credit reports.
Without transparency, there is a risk that consumers may be treated unfairly. Building algorithms that are explainable and fair is critical for maintaining consumer trust.
The social impact of credit scoring
Credit scoring systems can also impact social dynamics. Access to credit can influence individuals’ economic opportunities. Not receiving a loan can limit a person’s ability to achieve goals like buying a home or starting a business. Therefore, it’s essential to consider the broader implications of scoring systems on society.
As machine learning continues to evolve, stakeholders must remain vigilant about these ethical challenges. Collaboration between technologists, consumers, and regulators is vital to fostering an inclusive financial environment.
Future trends in machine learning for credit scoring
Future trends in machine learning for credit scoring are set to transform how lenders assess risk and make lending decisions. As technology advances, we can expect significant improvements in accuracy, fairness, and customization.
The rise of automated systems
One major trend is the continued rise of automated credit scoring systems. These systems utilize machine learning algorithms to analyze vast datasets in real-time, enabling faster and more accurate credit assessments. As automation becomes more sophisticated, it will enhance efficiency for lenders while providing consumers with quicker responses.
Improved predictive analytics
Another promising trend involves advancements in predictive analytics. Machine learning models will become better at forecasting borrower behavior by incorporating a wider range of data. Factors such as alternative data sources, including payment history from utilities and rent, are likely to be integrated into scoring systems. This inclusion can help provide a more complete picture of a borrower’s creditworthiness.
- Utilization of big data: Leveraging big data allows for more granular analysis of consumer behavior.
- Enhanced personalization: Lenders can offer tailored products based on individual scoring models.
- Dynamic scoring models: Developing models that adjust in real-time will reflect changing borrower circumstances.
As we look toward the future, ethical considerations will also be at the forefront. Ensuring that machine learning algorithms are fair and unbiased is critical. Ongoing discussions around transparency and accountability in scoring processes will shape the development of these technologies.
Moreover, regulatory bodies may impose guidelines to ensure that machine learning practices in credit scoring are responsible and just. Adoption of ethical AI frameworks will be essential for maintaining consumer trust in these evolving systems.
FAQ – Frequently Asked Questions about Machine Learning in Credit Scoring
How does machine learning improve credit scoring accuracy?
Machine learning analyzes vast data sets to identify patterns and trends, leading to more precise assessments of borrower risk.
What are some ethical concerns with machine learning in credit scoring?
There are concerns about bias in data, transparency in scoring processes, and ensuring all borrowers are treated fairly.
What role does alternative data play in credit scoring?
Alternative data, such as utility payments and rental history, helps create a comprehensive picture of a borrower’s creditworthiness.
What future trends can we expect in machine learning for credit scoring?
We expect advancements in predictive analytics, automation, and the incorporation of diverse data sets to enhance risk assessments.